Following Quadratic Programming with R, this is another example of how to solve quadratic programming problem with R package “quadprog“.
Why Another Example?

“quadprog” package requires us to rewrite the quadratic equation in the proper matrix equation as above. You may have the following questions:
- What if our equation is to maximize the objective function instead of minimize it?
- What if the inequality constraints are “less than (<=)” instead of “more than (>=)”?
- What if there is no equality constraints?
- …
To give you an idea how to answer these questions, this post will give you another example.
Example
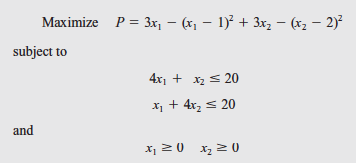
Be aware that this problem is to maximize the objective function and the first two inequality constraints are in “<=” form.
Therefore, we have to rewrite it as follows:
Minimize P = x12 + x22 – 5x1 – 7x2 + 5, subject to:
– 4x1 – x2 >= – 20
– x1 – 4x2 >= – 20
x1 >= 0
x2 >= 0
Matrix Notation
First, consider the matrix notation for a general quadratic function of two variables: x1 and x2:
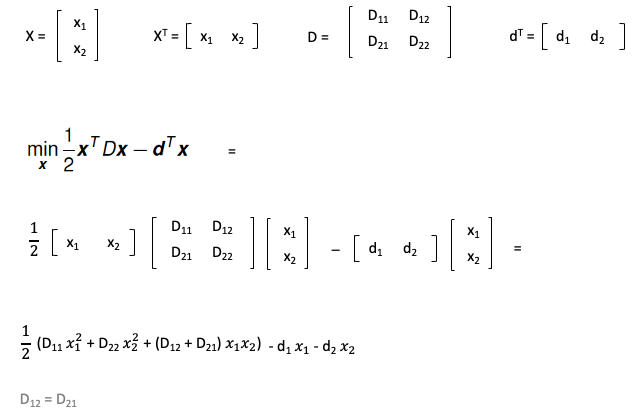
Second, we can extract the matrix D and vector d by parameter mapping to our example:

Similarly, the constraint matrix AT and the vector b can be found:
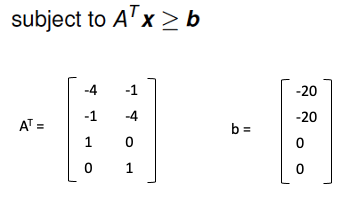
R Code & Output
solve.QP(Dmat, dvec, Amat, bvec, meq=0, factorized=FALSE)
- Dmat matrix appearing in the quadratic function to be minimized. - dvec vector appearing in the quadratic function to be minimized. - Amat matrix defining the constraints under which we want to minimize the quadratic function. - bvec vector holding the values of b_0 (defaults to zero). - meq the first meq constraints are treated as equality constraints, all further as inequality constraints (defaults to 0). - factorized logical flag: if TRUE, then we are passing R^(-1) (where D = R^T R) instead of the matrix D in the argument Dmat.
# Load "quadprog" package
# library(quadprog)
# Matrix appearing in the quadratic function
Dmat <- matrix(c(2, 0, 0, 2), nrow = 2)
# Vector appearing in the quadratic function
dvec <- c(5, 7)
# Matrix defining the constraints
Amat <- t(matrix(c(-4, -1, 1, 0, -1, -4, 0, 1), nrow = 4))
# Vector holding the value of b_0
bvec <- c(-20, -20, 0, 0)
# meq indicates how many constraints are equality
# No constraint is equality in this example, so meq = 0 (by default)
qp <- solve.QP(Dmat, dvec, Amat, bvec)
qp
$solution
[1] 2.5 3.5
$value
[1] -18.5
$unconstrained.solution
[1] 2.5 3.5
$iterations
[1] 1 0
$Lagrangian
[1] 0 0 0 0
$iact
[1] 0
Therefore, when x1 = 2.5 and x2 = 3.5 the quadratic function is minimized (-18.5 + 5 = -13.5, don’t forget the constant 5). However, since we rewrited the objective function at the beginning, we have to interpret the results accordingly. In other words, in order to maximize the original quadratic function, we choose x1 = 2.5 and x2 = 3.5. Further more, the maximized values is 13.5.
Hi Henry,
thank you so much for this Contents.
Can you explain how to solving portfolio optimization with value at risk measure in r?
Dear Henry,
thank you for the wonderful example.
What are some common operations performed using matrix notation, such as matrix addition, subtraction, multiplication, and transposition?
Dear Henry.
Thanks for the contents. It is great help to understand QP.
Epoxy Resin Pipes in Iraq ElitePipe Factory stands at the forefront of producing epoxy resin pipes, known for their exceptional durability and resistance to corrosive substances. Our epoxy resin pipes are engineered to meet the rigorous demands of various industries, offering a high level of performance in applications such as water supply, sewage systems, and industrial fluid handling. The advanced technology and high-quality materials used in our manufacturing process ensure that our epoxy resin pipes deliver reliable and long-lasting service. ElitePipe Factory’s dedication to innovation and quality makes us one of the most trusted and reliable suppliers in Iraq. Discover more about our products at elitepipeiraq.com.